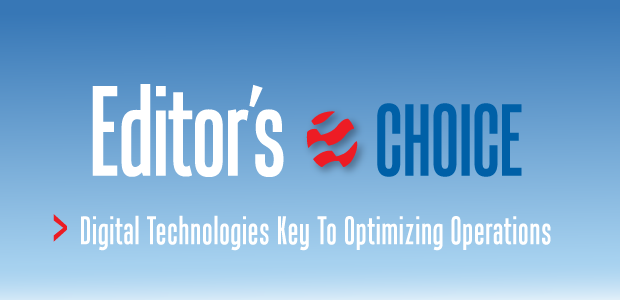
Digital Solutions Optimize Operations
By Sean FitzGerald
HOUSTON–Energy companies make consequential decisions every day that affect the future of their businesses. Producers take significant risks to find, drill, complete and produce oil and gas. Midstream companies invest billions to gather, process and send that energy to market.
While specialized applications can help to solve specific and complex problems in the industry, good decision making in select areas of the business is not enough to thrive in today’s market. Companies must execute in all areas of the business, on top-performing projects, with impeccable timing, and be ready to shift resources as market conditions change–all while continuing to operate their existing assets.
From upstream operators to pipelines that deliver products to world markets, informational value is the most important commodity throughout the oil and gas industry. Today, newly embedded devices and sensors make it easier to collect real-time data at many different touchpoints throughout the energy value chain, while cloud storage technologies make it simpler to store the data. Real value is created from data when informational context and associations are made inside and outside of an organization. However, making sense of the data is a complex process.
A GE study on digital technology applications found that only 3-5 percent of oil and gas equipment is connected digitally, and less than 1 percent of the data collected are used for decision making. There is clearly huge potential for market growth.
Market volatility provides proof that optimization is needed across the oil and gas value chain. Companies that leverage all the digital data available to optimize business processes will not only survive, but thrive during market downturns and dominate the industry on the next upswing.
To remain competitive, agile companies must make informed, data-driven decisions by leveraging a variety of computing tools and methods–from automated operations and intelligent field technology, to analytics and artificial intelligence. The variety of applications across the connected energy value chain share a common essential objective: extracting business intelligence from data to make better and timelier business decisions.
‘Connected Intelligence’
The key to optimizing enterprisewide upstream and midstream operations is the ability for companies to identify relationships, understand context and analyze data across the energy value chain as “connected energy intelligence.” Growth in the Internet of Things, or Internet-connected devices that collect and exchange data, makes connected energy intelligence possible. By leveraging the data from all areas of the oil and gas business with IoT and cloud computing–and bringing them together in new ways with analytics, big data and machine learning–these technology components form the basis for implementing connected energy intelligence.
The cloud and IoT go hand in hand, since the former enables the latter. The cloud delivers scalability on demand, without the fixed overhead of computing, storage and communication capacity. The result is ubiquitous computing, a world where computing cannot be distinguished between one thing and the next, or one decision to the next.
In 2015, nearly half of the drilling rigs in North America were idled in fewer than 12 months. Decisions were made during this time that shifted hundreds of billions of dollars as companies went from drill to survival mode. When the market shifts upward, how quickly will the industry be able to pivot? Cloud computing and IoT deliver the business agility and insight required by the oil and gas industry to quickly adapt to changing conditions.
In the modern energy workspace, the cloud and smart devices are making back-office tasks and field production operations ubiquitous through a unified open-source platform that automates workflows and integrates people, processes and systems across the entire value chain. The cloud and Internet of Things are hand in glove, allowing integrated business models to function more effectively.
The landscape for cloud and big data storage technology continues to change rapidly with new and improved technologies that leverage related software, many of which are open source. The use of open-source software makes the adoption of new big data technology economically feasible for the oil and gas industry. IoT involves more than simply deploying data collectors and collecting information in the cloud. Companies must also consider how the data streams will be ingested and stored for later consumption.
Data ingestion and integration considerations are akin to the midstream sector of oil and gas. Large, volatile flows of hydrocarbons must be conditioned and balanced into steady-flow products to downstream markets. The technology strategy must involve communication protocols, telecommunication limitations, stream analytics, and system integration. To satisfy these requirements, cloud platform offerings provide a toolbox of components, which when assembled together, offer a robust set of integration, protocol and storage options.
Data Integration
Integration platforms give companies the flexibility to change the format and destination of any piece of data at any time. Many companies struggle initially with uncertainty about where all the data will be stored, and this hesitation results in the failure to collect enough data to make analysis meaningful. Analytics platforms address this problem by enabling connectivity between disparate data sources, making it unnecessary to choose one (and only one) database technology like building a single enterprise data warehouse. This approach is simply not required anymore.
Data acquisition, storage and integration are links along the energy information value chain. Data models define the relationships between many structured and/or unstructured data elements. It is important to understand that data models can, and should, change as new discoveries are made. Yet, they do have to start with a project and a reason to justify their creation. Adequate documentation around the model and the metadata around each data element should be at the core of any new project. Models should be built around each area of the business through a process of:
- Defining the project;
- Collecting the data;
- Analyzing and evaluating the data;
- Building a model; and
- Deploying the model.
Today, most companies can gain tremendous benefits from connecting data silos, providing visibility into the basic relationships that are already known to exist, such as vendor and cost. It is not surprising that technology leaders over the last decade dominate in machine learning and artificial intelligence platforms. IoT and the potential value created by the petabytes of data streaming from billions of these devices is why these leaders are advancing the platforms and tools to eliminate the barriers to entry into this cutting-edge field.
Finding, drilling, completing and producing oil and gas wells is capital intensive. Big data and analytics are being leveraged as building blocks for enterprisewide upstream and midstream activities. By integrating data from all areas of oil and gas operations with IoT and cloud computing–and bringing them together in new ways with analytics, big data and machine learning–these technological components form the basis of a connected energy intelligence business model.
Unified Value Chain
The ability to automate, integrate, collaborate and optimize operations through a single unified value chain that integrates innovative solutions based on an open standards-based platform is the key to empowering oil and gas companies to conquer complex business challenges across the entire value chain, from land acquisition to the back-office.
From the beginning of the land acquisition phase in a new basin, companies must efficiently document, catalog, prioritize and value their leaseholds and mineral rights. As market conditions change, exploratory wells are tested and field development begins, creating “data silos” that are difficult to overcome in many corporate software ecosystems.
With disparate and disconnected systems, market volatility masks the problem when prices increase and leaves executives scrambling for answers when prices rapidly decline. There are many analytical and data services available that utilize public and internal sources of data to provide visibility to land availability and drilling activity.
Once the assets have been acquired and field development begins, a real-time field development engine continually can evaluate and refine reserves, revalue assets, and even alter development plans as conditions are met and milestones achieved. In the world of IoT, data streams are already available that provide each department with the real-time data it needs, but rarely provide value to any other organization unit.
During the land acquisition phase in a newly discovered basin, there is often an emotional component to land deals, and time is typically the only means of placing true value on each deal. Through artificial intelligence technologies such as cognitive and natural language interpretation, all emotion can be removed by applying an objective score. Scoring can be done by gathering inputs, scanning the lease with a mobile device, and processing the content of each page gathered.
When commodity prices drop rapidly, companies that make better and faster decisions will be positioned to widen the gap between them and the rest of the market, especially when capital budgets get cut. With the scoring system in place, connecting the dots to identify assets with the lowest objective score should be the first data sent to the data room. Data produces information that can be used to provide the acquisition and development roadmap given current and expected market conditions.
Drilling And Completions
Finding, drilling and completing oil and gas wells is extremely capital intensive. As companies strive to improve production output and extend the life of their assets, it is not surprising that big data and analytics already are being leveraged to optimize these core activities.
Data models use hundreds, sometimes even thousands, of controllable inputs: drilling location, depth, fracture stages, lateral length, proppant type and amount, pump pressures and rates, etc. The drilling process no longer consists of a tool-pusher capturing drilling depth on a paper sheet. Data streaming is the “new normal,” where fiber optics are used to sample hundreds of sensors every few seconds and gather rates, pressures, weight, torque, molecular composition of gas captured, etc. It is now common for a drilling engineer in Houston to remotely monitor drilling activity in Pennsylvania.
Companies that continue to push for improvements are building their field development plans and designing their drill sites and completions long in advance, often using predictive analytics. If the wrong decision is made while the bit is in the ground, additional costs and delayed revenue are the most that companies can hope for out of a well, while the worst case is a complete loss. Yet, companies typically have more than enough data to drive better decisions. Companies with a culture of continuous improvement through analytics find that integrating 3-D seismic data with completion designs improves the long-term economics of every well drilled by increasing production and reducing the risk associated with well design.
Production Operations
For a company to succeed in optimizing production operations, oil and gas production data must be used on a day-to-day, hour-by-hour basis. Technology and information have been lockstep with capital expenditures for decades, but production operations and the corresponding operating expenses largely were ignored.
The ability for companies to collect, process and analyze production and operational data never has been easier. Oil and gas producers can make incremental improvements that compound over the entire productive life of a well. The omission of real continuous improvement strategies during production is why most leading experts and technology innovators are particularly focused on production optimization that uses process control automation and preventative equipment maintenance.
Production optimization is not solely dependent on producing more oil and gas all the time. To truly optimize production, companies must produce smarter with less overhead. Efficient companies already have introduced mobility into every aspect of operations. Accuracy is a side effect of manual data collected at the source using smart devices such as tablets. However, capturing a set of important data points once a day by sending a field technician is incredibly inefficient.
Utilizing devices and sensors to capture pressures, rates and equipment control data on high frequencies yields far more accurate datasets, improving an engineer’s ability to make production decisions. The scarcity of engineering knowledge increases the time between receiving event information and a decision’s positive outcome or negative impact. Intelligent systems must also deliver information back to the field technician, providing actionable information rather than just a better means of collecting data.
According to a McKinsey & Company report on digitizing oil and gas production, automation and optimization will yield the most substantial results for any upstream company. Process control automation of oil and gas assets has been a part of localized production operations for decades from the perspective of a well, facility and platform driven by safety and maximizing output. With the limiting factors of computing and storage infrastructure removed, the industry can move beyond observational analysis to continuous improvement via data models with many input variables associated to data points sourced outside of the production system.
Back-Office And Midstream
Back-office cost centers of oil and gas companies will become the controllers of innovation in the future, because they sit in the middle of big data for the oil and gas industry and they put a dollar value on all of it.
It is often surprising how much effort goes into keeping the data moving between silos. Data models exist in industries such as retail and manufacturing that are very similar to what energy companies require. Energy producers need to manage inputs through vendor performance and optimize output through hedging and risk management, the same way an automobile manufacturer must manage its suppliers and the demands that are influenced by commodity markets.
Hundreds of vendors are used to locate, drill, complete and produce oil and gas, which is very similar to a manufacturer. The energy industry is built on relationships and an assumption of value. Commodity downturns test the business relationships between producers and service providers as every invoice is scrutinized at a granular level. Decisions made on relationships alone are why producers can achieve immediate efficiency gains through service provider cost reductions. Like manufacturing, a big data solution can identify performance and quality trends of every vendor, allowing decisions on the merits of quality and reliability rather than cost or location.
Companies engaged in the midstream operations suffer from many of the same operational issues as upstream producers, such as downtime caused by equipment failures and field logistic inadequacies. Unconventional production growth has added volatility to one of the more stable segments of the energy industry as producers expand in new basins not equipped for massive increases of natural gas and liquids production.
Consider a natural gas gathering and processing company with operations in a rapidly expanding basin. New plants are being constructed, compressor stations are brought on line, sales meters are installed at each well pad, and everything is connected through a supervisory control and data acquisition system. The device and sensor data also are streamed to a large, big data analytics platform that also is connected to the back-office enterprise resource planning solution with measurement, contract, financial as well as commodity price data. The company also has worked out terms with their trading partners on data enrichment agreements that allow for information to flow between organizations.
Since companies in this sector make the most profits through maintaining a consistent flow of product at a target capacity, big data solutions have application value. In many cases, it is simply not possible for a single human being to process, analyze, interpret and interpolate where and how to adjust flow rates and send those notifications and control alerts to their upstream customers every hour of the day. By tapping into real-time data streams from remote devices and sensors, and applying machine learning, opportunities can be identified that improve the flow of gas through the system, resulting in more favorable terms for customers.
As connected systems for oil and gas expand through the cloud, with data flowing seamlessly from drill bit to burner tip, upstream production activity can account for changes in market conditions and energy demand without any human intervention.
Additional opportunities for improvement are available through the application of cloud computing, machine learning and predictive analytics against continual ingestions of data that affect the results of each model. Cloud computing encourages the efficient use of capital while expanding computing and storage capacity. And with today’s capabilities in machine learning, companies can expand beyond one scenario that engineers and management conceptualize, and instead run through many potential scenarios to produce the best path forward.
Companies will continue to have better access to data from their trading partners and service providers, including devices in the field that stream real-time production data, real-time market and commodity pricing, and risk predictive models. Strategically levering all the digital data available will play an important role in the decisions that executives make to navigate their organizations through the ups and downs.
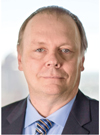
Sean FitzGerald is vice president of engineering at Quorum, with primary responsibility for overseeing the development and maintenance of the company’s software applications. With 20 years of software engineering experience, he served in leadership positions at Diablo Management Group, Visual Numerics, Rogue Wave Software and X-ISS prior to joining Quorum. FitzGerald holds a B.S. in information systems and an M.B.A. in information technology management from the University of Athabasca.
For other great articles about exploration, drilling, completions and production, subscribe to The American Oil & Gas Reporter and bookmark www.aogr.com.