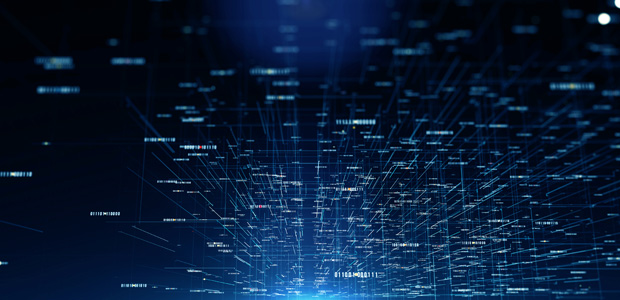
Big Data Analytics
Platforms Optimize Engineering Workflows
By Amit Mehta
HOUSTON–Are big data analytics really important in the oil and gas business? The answer is resoundingly “yes” according to recent polling of industry executives, with one report stating that four of five executives in the C-suite believe that big data analytics is one of the top three corporate priorities.
But in an era that heralds the power of big data, artificial intelligence, cloud computing and other technologies, it is still human beings that make critical business decisions and drive a company’s competitive advantage. The right big data analytics solution can help oil and gas professionals make better and faster decisions, and sharpen their organizations’ competitive edge.
In the world of big data, experts often refer to the five Vs of data: velocity, volume, value, variety and veracity. There is sometimes a misconception about the highest importance for successful execution of big data solutions. The digital revolution is giving operators a new appreciation of the value of their data, and a new understanding of data as an asset. This has led oil and gas companies to hire data scientists and analysts, all of whom provide different rankings of which V is more important based on their experience and expertise.
From the perspective of experts at Gartner Analytics and Silicon Valley, variety is the most important V in big data value equation. However, what does variety really mean? In the workplace, variety means machine data (structured data sitting in a variety of forms and applications); human data such as photos, videos and human decisions; “black data” that was never assumed to be of high importance; and a combination of all the above located in a variety of corporate databases. All this information has to be consumed automatically as raw data, cleaned on the fly, and then run against advanced machine learning and artificial intelligence algorithms to create actionable insights.
For oil and gas drilling, completion and production engineers, big data analytics platforms augment human intelligence and skillsets by instantly providing all actionable insights, which they can use as the ground truth of their decision making workflows.
Analytics Triangle
From a working perspective, the big data analytics triangle is comprised of business analytics, workflow analytics and technology analytics (Figure 1).
Business analytics are focused on leveraging big data to create business forecasts, business analysis, advanced pricing models, etc. A good analogy is how credit card companies mine a variety of consumer data to develop new offerings.
Workflow analytics leverage big data to optimizing “as is” enterprise workflows to enable zero-decision latency. A good analogy here are the platforms developed by the likes of cell phone companies, Amazon and Uber. The variety of big data involves leveraging structured (machine) and unstructured (human generated) data to help simplify consumers’ daily lives and create customer reliance in doing so.
Technology analytics use big data to optimize hardware (i.e., machine data only) to make downhole tools much smarter, thereby creating a technology edge via innovation. Examples include potentially using robotics on rigs, predicting downhole tool failures, and offering new advanced completion tools and intelligent plunger lift systems.
Many energy industry big data projects today seek to apply machine data (technology analytics) in a capital-intensive market. This makes sense given the industry’s significant hardware infrastructure. Understanding the value of analytics/predictions to predict outcomes from machines/hardware has a natural bias and is probably easy to quantify. Hence, the industry’s instinctive focus is to implement machine data projects first.
The problem with taking this approach is that it has obvious tangible short-term benefits as engineers understand the problem domain well, but it does not result in an integrated growth platform in the long-term. Furthermore, if it is built in-house leveraging self-service offerings of big data vendors, companies risk being in long execution cycles and losing the competitive edge.
Growth Platform Principles
Looking at lessons learned from successful Silicon Valley big data implementations, many successful big data initiatives were built on the sound principles of a growth platform that:
- Supports a diverse set of participants and offers opportunities for creating value in many distinct areas;
- Scales up by accommodating a large user base without eventually adding unacceptable costs or issues;
- Generates increased returns as participation grows;
- Evolves continuously in functionality over time, providing incentives for participants to engage regularly and share learnings;
- Provides development leverage (investment required to build additional functionalities) and interaction leverage (effort and cost for a diverse set of participants to facilitate interaction); and
- Defines practices to eventually guide the activities of a large number of participants in the enterprise.
The immediate challenge for oil and gas companies looking to leverage big data analytics is figuring out where to start. A logical first step is to assess key learnings from successful big data implementations in other industries.
For example, one technology company opted to focus on the workflow analytics approach as the first order of business in building a foundation for an integrated growth platform in partnership with its clients. Notably this was not an easy approach since it required heavy lifting upfront to uncover user habits, and then change habits of working for years in applications such as Excel™ or home-grown systems called building platforms. Inherently, the problem with these systems is that the knowledge/expertise is limited to few resources, and the systems do not comply with growth platform principles and only grow more cumbersome over time.
Fortunately, this problem was addressed by building a decision platform utilizing the sound principles of a ready-to-use growth platform. The platform creates insights from a variety of data (machine and/or human) to enable zero decision latency, so that engineers can act on the insights (workflow analytics) by amplifying their knowledge.
Taking another example from outside the industry, many consumers have ordered transportation services from the Uber phone app, which offers multiple attributes. Think about it. Uber can combine data (variety) into actionable insights that tell the customer what a service request will cost and what time the vehicle will arrive at their location. There is no data scrubbing, data manipulation or guessing about costs, because the information is right there for the consumer to act on.
A Houston-based technology company took the same approach to ensure it could help simplify engineers’ workflows, similar to what Uber has done for riders. It resulted in reducing complexity in “as is” workflows, which brought agile decision making, and agility ultimately resulted in substantial operational efficiency improvements in engineering projects.
Creating Customer Intimacy
The real work comes in creating customer (user) intimacy, which involves change management. Deeper customer intimacy involves starting with bigger questions, such as how big data analytics solutions can make the life of an enterprise user easier and improve their workflows, similar to what consumer products do in daily life. It is important to understand usage patterns to drive stakeholder engagement throughout an organization, have the ability to make real-time modifications to a solution based on customer feedback and scale up by accommodating a large user base without adding unacceptable cost. Above all, a solution must be able to satisfy the needs of enterprise users quickly.
Deeper customer intimacy is not a traditional strength of independent software vendors. Implementing effective big data solutions can challenge old ways of thinking and require developing new skillsets. For instance, one independent software vendor adopted manufacturing principles to software development by treating daily user needs as a raw material to be converted to a finished good (user idea live on platform) in weeks rather than months or years.
The company has invested heavily in data quality to ensure decision insights on its platform are accurate since that is what matters most to enterprise users. It has focused its efforts on mapping “data to decision” loops for enterprise users and has significantly reduced latency in them to provide accurate, actionable insights to the user.
Moreover, the software vendor has adopted the mindset that each enterprise user is a consumer who uses data daily to make wide-ranging decisions. The company recognized that a platform will be doomed if the user cannot make accurate decisions instantly. Ultimately, a platform must be able to easily integrate the point applications in technology and business analytics dimensions, since they solve specific problems and have a role to play.
Companies must realize that identifying, capturing and delivering on opportunities more quickly than their competitors requires breaking old workflow patterns and operational paradigms. They must modify rules of the game and update their playbooks to ensure they can be “right in time,” or risk themselves and their organizations. This requires balancing defensive and offensive execution of big data, which can be evolved with time to avoid becoming another failed experiment.
With the right approach, companies can synchronize business and/or technology big data analytics projects with workflow analytics to build a successful integrated and agile growth platform.
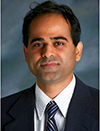
AMIT MEHTA is chief executive officer of Houston-based Moblize, which offers integrated analytics platforms for oil and gas engineering workflows. Mehta holds bachelor’s and master’s degrees in manufacturing and management from the University of Cambridge.
For other great articles about exploration, drilling, completions and production, subscribe to The American Oil & Gas Reporter and bookmark www.aogr.com.